Adresse
Infodoc : Réseau des bibliothèques et centres de documentation d'AgroParisTechFrance
contact
Array ( [TITRE] => <b>Type de document : </b> [TITRE_CLEAN] => Type de document [OPAC_SHOW] => 1 [TYPE] => list [AFF] => Livre [ID] => 4 [NAME] => cp_typdoc [DATATYPE] => integer [VALUES] => Array ( [0] => 5 ) )
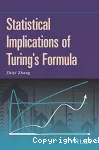
Titre : |
Statistical implications of turing's formula
|
Auteur(s) : | Zhiyi Zhang, Auteur (et co-auteur) |
Type de document : | Livre |
Sujets : | Statistique |
Résumé : |
Turing's formula is, perhaps, the only known method for estimating the underlying distributional characteristics beyond the range of observed data without making any parametric or semiparametric assumptions. This book presents a clear introduction to Turing’s formula and its connections to statistics. Topics with relevance to a variety of different fields of study are included such as information theory; statistics; probability; computer science inclusive of artificial intelligence and machine learning; big data; biology; ecology; and genetics. The author provides examinations of many core statistical issues within modern data science from Turing's perspective. A systematic approach to long-standing problems such as entropy and mutual information estimation, diversity index estimation[...] Turing's formula is, perhaps, the only known method for estimating the underlying distributional characteristics beyond the range of observed data without making any parametric or semiparametric assumptions. This book presents a clear introduction to Turing’s formula and its connections to statistics. Topics with relevance to a variety of different fields of study are included such as information theory; statistics; probability; computer science inclusive of artificial intelligence and machine learning; big data; biology; ecology; and genetics. The author provides examinations of many core statistical issues within modern data science from Turing's perspective. A systematic approach to long-standing problems such as entropy and mutual information estimation, diversity index estimation, domains of attraction on general alphabets, and tail probability estimation is presented in light of the most up-to-date understanding of Turing's formula. Featuring numerous exercises and examples throughout, the author provides a summary of the known properties of Turing's formula and explains how and when it works well; discusses the approach derived from Turing's formula in order to estimate a variety of quantities, all of which mainly come from information theory, but are also important for machine learning and for ecological applications; and uses Turing's formula to estimate certain heavy-tailed distributions. |
Editeur(s) : | John Wiley & Sons |
Date de publication : | 2017 |
Format : | 282 p. / réf., Index auteurs., Index sujets. |
Langue(s) : | Anglais |
Identifiant : | 978-1-119-23706-8 |
Lien vers la notice : | https://infodoc.agroparistech.fr/index.php?lvl=notice_display&id=194226 |
Exemplaires (1)
Localisation | Emplacement | Pôle | Section | Cote | Support | Disponibilité |
---|---|---|---|---|---|---|
Kourou | MATHEMATIQUE - STATISTIQUE - INFORMATIQUE | AgroParisTech-Kourou | F104.ZHA.2017 | Papier | Empruntable Disponible |